How to Reduce Uncertainty in Product and Startup Traction
Simple Frameworks from startups, growth hackers, software engineers, and more that help you make better decisions.
.png)
Hi. I’m Chris and I’m a frameworks junkie.
I’ve been addicted to frameworks, techniques, and methodologies for more than 25 years. In fact, my passion for voracious learning started shortly after I left school. (Which was unfortunate timing for my high school grades.)
If there is one domain in which I’ve ravenously consumed every framework ever created, it is anything and everything related to startups and product growth.
So what happens when Mr “I’ve got a framework for that” suddenly encounters a string of issues for which there are no playbooks or frameworks to solve?
This happened to me in 2018 when I was Chief Marketing Officer at Topology Eyewear - a highly unusual startup that made custom eyewear from scratch based on a scan of a person’s face from their iPhone. At a certain point, we started needing to make decisions for which there were no frameworks, no books, nor even blog posts.
After much anxious and fruitless searching for models or case studies to help, I realized something more foundational: There was no precedent for this because nobody had done this before.
💡 The path never trodden is always uncleared.
When I realized the answer was fundamentally unknowable, I turned to our CTO, Alexis Gallagher for advice. By his own admission, Alexis knew nothing about marketing or growth, but I had noticed that he had a way of reasoning towards good decisions even in the face of great uncertainty. How did he do this?
Alexis explained to me over lunch the basics of logic and reasoning, then directed me to several books to learn more, including
- The Book Of Why - Which I found virtually impenetrable, especially as an audiobook.
- Thinking Fast & Slow - Which was fascinating
- Thinking in Bets - Annie Duke
As fascinating as the content and insights were, the main realization I took from studying these books was the enormous overlap with some of the existing startup techniques, and the opportunity to augment startup & marketing techniques with decision-making techniques.
Another 6 years later, I now believe that decision-making in the face of uncertainty is one of the most important meta-skills that any startup or product leader needs to learn. Because the more innovative your product or startup is, the less likely someone else’s anecdotes and case studies will apply to you.
This also makes startups the ideal domain from which to draw insights to help decision-making techniques in other domains.
So in this post, I am delighted to partner with the good folks at The Uncertainty Project to offer a collection of frameworks drawn from the diverse worlds of startups, marketing, growth hacking, software development, and more.
Read on to find simple methods to help you get unstuck the next time you are doing something for the world’s first time.
Startups are Defined by Uncertainty
In his bestseller The Lean Startup, Eric Ries defined a startup as:
A human institution designed to create a new product or service under conditions of extreme uncertainty
Those last two words are the most important. The presence of extreme uncertainty is what distinguishes a startup from a regular "small business."
Eric goes on to credit uncertainty to be his main motivation for writing the Lean Startup:
“because most tools from general management are not designed to flourish in the harsh soil of extreme uncertainty in which startups thrive.”
So after 8 years of experimenting with various decision-making techniques in the punishing environment of start-ups, these are some principles and techniques I’ve found to be helpful.
Everything is an assumption
One of the first lessons from Steve Blank and Eric Ries is that everything you think you know about your startup is probably wrong, so your job as an entrepreneur is to systematically discover where you are wrong and adapt accordingly.
This insight gave birth to concepts such as “Leap Of Faith Assumption” (LOFA) and “Pivot” that have since transcended startups and become standard parlance within most modern businesses.
Do the Riskiest Thing First
In her recent book “Quit” Annie Duke tells the stories of the California Bullet Train and Astro Teller’s “Monkeys and Pedestals” framework to teach the importance of working on the riskiest part of a project first.
Simply put, there is no point in working on the easy or known part of a project while leaving the unknown part untested. If the unproven aspect resolves to be unfeasible, all the effort spent on the easy part is wasted.
While this is true in any project or endeavor, it is especially critical for startups, because startups have a disappearing “runway.” Each injection of startup funding starts a countdown by when the startup must take off, or go bust. Such impending doom is much more visible and visceral in a startup than in a mature business, where project timelines are more fluid. This is why startups, and especially tech startups have techniques like “spikes” and MVPs built into their DNA.
As David Bland said in the first episode of his podcast “How I Tested That” in which I was a guest:
You can’t pivot if you have no runway left
Tool To Try: Assumptions Mapping
So how do you know which assumption is riskiest to test it first?
As the Author of “Testing Business Ideas” and a career expert in idea validation, David is known for a simple but effective method to prioritize assumptions for testing, which he calls Assumptions Mapping.
Assumptions Mapping divides risk into two factors:
- Importance: If we are wrong about this assumption, how much does it matter?
- Evidence: How much proof or evidence is our assumptions based on?

By mapping each assumption to this chart, the priorties emerge as the assumptions that are most critical for the business, but for which we have the least evidence.
Watch David’s overview to learn how to apply it for yourself.
Everything is a Bet.
Fans of Annie Duke will be familiar with her advice to frame assumptions in the form of a bet, such as you would when playing poker for example.
To place a rational bet, you must quantify both the size of the potential prize, and the likelihood of winning. Even without placing a real wager, challenging yourself to try and answer these points helps you make a decision based on “expected value.” Or perhaps realizing that you can’t quantify the numbers reveals that you’re not ready to make a decision yet.
Betting aside, here are a few other techniques from the worlds of tech and startups that also challenge and help you quantify uncertainty, in order to escape it.
Tool To Try: Hypothesis Driven Development
Here’s a technique I’ve long considered to be under-rated, or just undiscovered: HDD.
I first learned of HDD from Barry O’Reilly’s post for Thoughtworks. The article explains the approach of forming any given feature or experiment in particular format:
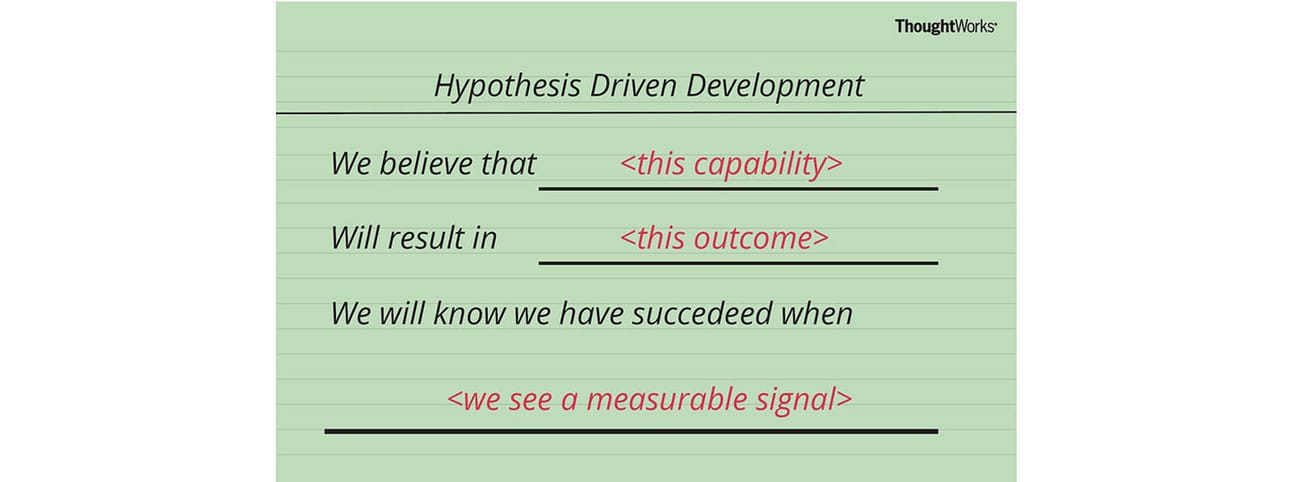
Like Annie’s framing of placing a bet, The first benefit of this method is that it forces you to consider whether something could be sufficiently valuable at all. Try it for your next project and you might be surprised to find how hard it is to state any kind of assumption about what the upside could be.
Tool To Try: Growth ICE ranking
The HDD format of experiment framing works great when combined with another tool for ranking growth experiments.
Growth marketers, often referred to as growth "hackers," typically compile dozens of ideas aimed at improving customer acquisition and retention. They also require tools to prioritize which experiments to conduct next.
A popular ranking methodology presented in the book "Hacking Growth" scores an experiment idea based on three factors.
- Impact: How big could the positive impact of this idea believably be?
- Confidence: How sure are we that the impact can be realized?
- Ease: How cheap/fast/simple is it to implement the idea?
By adding these factors, and ranking the sheet by their sum, we start to see which experiments are likely the most valuable to start with.

Notice that the framing of “confidence” really grounds the method in terms of uncertainty, and the outcome similar to expected value. Unlike other marketing ranking frameworks, ICE is predicated on all experiments being guesses, and all impact being uncertain.
Practical tip:
When you start using this model, you soon find that the scoring process is more relative than absolute, which can get messy. So it helps to develop some internal definitions of what qualifies different scores. For example, I have developed my own scoring for confidence as follows:
- High (7-10) To score an idea as high confidence, we’d need prior evidence that this idea has worked before. A maximum score would mean we’ve proven it works for the same product, brand, and customer.
- Medium (4-6) This might be an idea that we have not tried before for our product or brand, but there is precedent that it works in other relevant companies. Perhaps a team mate has successfully implemented it at a previous company, for example
- Low (1-3) This is a new, speculative idea with little or no precedent to prove that it would work
You Never Have “No Idea”
As soon as you try advance ranking experiments and ideas in this way, you will quickly run into issues where you feel you “have no idea” what size of the impact could be.
The good news, is that you DO have SOME idea, and with help of - yes - another technique, you can guess closer than you think to the right answer.
Tool To Try: Use a Fermi estimate, Subdivide, Then Benchmark
A Fermi estimate is named after the Italian-American physicist Enrico Fermi, who famously used the method to approximate impact of the first atomic bomb during the Manhattan Project.
I first learnt of this technique from the book “Scaling Lean” by Ash Maurya, where he explains the efficiency of estimating using orders of magnitude, to approximate how many piano tuners there are in Chicago. The way I like to explain it is in estimating something big, like the wingspan of a 747 Jumbo Jet, which I genuinely do not know.
For example:
- What is the wingspan of a jumbo jet?
- I have no idea
- Is it 1 meter?
- No, bigger
- Is it 10 meters?
- No, bigger
- Is it 100 meters?
- Hmmm, I don’t know, but I think it is smaller than 100 meters.
Already it is possible to see that I do have SOME idea. It is somewhere between 10 and 100 meters wide. But I can safely go further by starting to bisect those orders of magnitude:
- Is it closer to 10 meters or 100?
- Defintely closer to 100
- Is it closer to 50 or 100 meters?
- Hmm. not sure, but I can visualize 100 meters as the straight on an athletics track, or 50 meters as an Olympic sized swimming pool.
- Imagining a plane sitting on top of each of those, I am not sure, but I guess it is closer to the pool, maybe a little larger.
- Therefore I’m going to estimate about 60 Meters.
(Checks answer on Perplexity…)

I promise you I just did that in one pass, realtime, without cheating! From “no idea” to 93% accuracy in less than a minute.
So next time you find yourself with “No idea” of how to size the impact of an uncertain scenario, try this trick and discover that you actually do.
Growing Fast and Slow
Once you’ve plunged into the experiment rabbit hole, and start reading more, you’ll soon encounter the experimentation velocity argument.
Many argue that since the goal of a startup is to experiment and de-risk unknown assumptions, then experimentation itself is a leading indicator of success. Therefore, the argument goes, experimentation velocity (n of experiments per week/month) is the most important north star metric for a startup or growth team.
Experience has taught me to disagree with this.
Learning Velocity, Not Experiment Velocity
When you start to optimize for experiment velocity, you start churning out poor experiments. And there is no slower path to learning, than wasting even small amounts of time on experiments that you learn nothing from. Instead of tracking velocity of experiments, you should be tracking velocity of validated learning.
In 2019 I delivered a talk at a Growth Marketing seminar in San Francisco called “Growing Fast and Slow.”
Taking the stage right after the great Guillame Cabane, I argued that experiment velocity is faux optimization, and reducing throughput of experiments can actually lead to faster progress.
The name of my talk was obviously inspired by Daniel Kahneman’s “Thinking Fast and Slow” which I had just read. What I learnt from that book and reflected in my lecture was that in startups and growth teams we often reflexively jump straight to creating an experiment without bothering to using our slow brains to properly appraise whether we need to test at all.
💡 The slowest learning velocity comes from running experiments you don’t need to run at all
The First Source of Waste is Conducting Experiments Whose Results You Don't Trust
In theory you’d think you would never fall into this trap, but in practice it happens all the time.
You run an experiment, get a result, but then rationalize away the result by saying, “Ah, but of course those customers chose that way, because they were unfairly influenced by XXXX”
This is why I recommend laboring over experiment design. Not because I’m a perfectionist, but because I don’t want to waste time and resources getting data that I won’t even trust myself.
Better to slow down and plan an experiment to create trustworthy data, than rush to create unreliable data.
Avoid Redundant Experiments
What if you don’t need to run the experiment at all?
In an old Medium article I wrote to accompany the talk, I described 5 different types of wasted experiments, of which one is when you your experiment delivers a completely obvious answer. Of course some things only seem obvious by hindsight, but oftentimes they were also obvious in foresight, if only we’d to estimate the outcome.
Tool To Try: Experiment Premortem
The concept of a "premortem" was created by Gary Klein as a strategy in decision making.
It is designed to help teams identify potential problems in a plan or project before they occur. By imagining that a project has failed and then working backwards to figure out what could lead to this failure, teams can proactively address and mitigate risks.
In this case, the idea is not only to ask why something failed, but to imagine the possible variable outcomes from the experiment, (such as win, lose, draw) and ask yourself why they happened. The act of doing so sometimes unveils forces that would invalidate your experiment, or be so unsurprising that you need not run the experiment at all.
Irrelevant Consequences
Now that you have mapped the possible variable outcomes from an experiment, you can avoid the next form of wasted experiment - one that wouldn’t change your actions anyway.
This is where you run an experiment to discover an unknown, but the learning doesn’t actually change what you do as a result.
Case Study: Topology
When trying to unlock DTC growth in an early stage of Topology’s life, we experimented with whether asking or forcing customers to order a prototype of their glasses before ordering the final product would lead to higher overall conversion.
We imagined 3 different funnel paths that could work for us:
- A. Customer pays $10 to receive a prototype of their glasses
- B. Customer pays full price and proceeds Straight to Final order
- C. Customer gets to choose Optional Prototype OR Straight to Final
We felt did not know which the users would prefer, or what the economic returns of the optional prototype route would be. So we designed a thoughtful experiment, which would require:
- A few days of product design to change the UI
- A two-week sprint cycle to develop and release the software
- 5 figures of media spend to get enough audience exposed to the test
- At least 10 days of experiment duration to give time for results to mature
All in all, it was an expensive experiment, but we’d pre-modeled it to avoid the previously described forms of waste and we were excited to see what benefit it could drive.
But then we asked ourselves a new question: What if A won the experiment? What would we do next?
The first realization was that the threshold for victory was not the same for all 3 variables. For example the cost of making prototypes far exceeded $10, creating a loss on every prototype order. So A would need to “win” by a much higher threshold than B or C for us to consider implementing it for the future.
Then we realized something even more important: There was virtually no believable way that A would ever achieve that result. Then we looked again at Option B and realized the same was true. We already knew it could not win by the margin it would need to for us to choose it.
💡 Regardless of the experiment's results, our actions would have remained the same.
So we decided to stop all work on the experiment, and just apply the state of C in the app and go with it.
The results were immediate and game changing. And those extra few hours of modeling-out the outcomes and subsequent actions meant that we both saved the cost of the experiment AND delivered the growth to the business 4 weeks earlier.
Tool To Try: If This, What Next?
Write out the potential outcomes for an experiment (A, B, C) then for each possible outcome write down what you would do if the experiment did return that result.
What you might be surprised to find is that sometimes, all results lead you to do the same next action anyway, and so what is the point of running that experiment at all?
Just get on with it!
Uncertainty in Traction Design
My accumulated experience with uncertainty in product innovation is a key factor driving the need for the new methodology I call Traction Design.
In summary, we now work in an age where the feasibility (can we build it?) and desirability (Does anyone want?) of a product or startup are no longer the most uncertain or risky aspects. Thanks to the democratization of technology, design, and communication, we’ve never seen more great products launched. Instead, most products and startups die on the battlefield of traction.
So if, as we said earlier in this article, your job is to eliminate the riskiest uncertainty first, then validating traction potential should surely be the first place to start. Yet this is the opposite of how most startup and product teams operate when they spend 70% of their runway working on the product.
That’s why I’m developing the new methodology of Traction Design.
I believe that an idea for a product or startup has an existing level of “potential traction” that exists before the product is created. Counter intuitively, the traction of a product can be increased by factors outside of the product, before you even build the product.
Clearly there is a tension here. On one hand, I’ve said that startups are assumptions and you don’t know until you test. But as I’ve also written, some uncertainties can be resolved to an actionable extent with some slow thinking, instead of an experiment.
Both cases are true. The point is that most teams don’t even try either, and only find out they need to pivot when they have no runway left to do so.
I find it an unacceptable travesty that potentially great innovations die needlessly on the battlefield of traction because of this, and I don’t accept there is nothing we can do about it.
____________________________________
Chris “Guesto” Guest is a 3-time start CMO turned advisor specializing in Lean Category Design, positioning, and Traction Design. A Brit living in the San Francisco Bay Area, Chris has almost 25 years experience leading marketing for some of the world’s most prestigious tech brands such as Audi, Ferrari and McLaren, as well as startups across North America and Europe. Chris also founded the North Bay (Marin & Sonoma) chapter of The Gen AI Collective and writes the Traction Design publication on Substack.