Building a Belief Portfolio
In strategic conversations, there is a lot that remains just below the surface.
The mental models that each person brings to the table shape the dialog, discussion, and debate, yet they are rarely transparent. What if we could make them transparent? How would that be different?
“To state your beliefs up front - to say, ‘Here’s where I’m coming from.’ - is a way to operate in good faith and to recognize that you perceive reality through a subjective filter.” - Nate Silver, “The Signal and the Noise”
To “operate in good faith” then, it is important to expose the beliefs that shape and filter the “sense-making” across the leadership group. But this isn’t a natural behavior, and it rarely takes place, unless a structured approach in introduced.
Why does this matter?
- In sense-making, our beliefs (based on experience and expertise) are the “why” behind our perceptions and choices
- With the pace of change, we need to be able to easily re-evaluate our beliefs, when new information becomes available
- With a diverse set of expertise and experience across the organization, there will be many different beliefs, and we want to be able to leverage this as an asset
Overall, an emphasis on surfacing, documenting, and re-evaluating shared beliefs can bring a stronger foundation to the strategy table.
“Part of the skill in life comes from learning to be a better belief calibrator, using experience and information to more objectively update our beliefs to more accurately represent the world. The more accurate our beliefs, the better the foundation of the bets we make.” - Annie Duke, “Thinking In Bets”
Documenting Beliefs
In an organization, we are constantly scanning and surveying our environments, internal and external, to shape opinions about the (unknowable) future. These are often external circumstances that are outside our direct control, yet have huge implications on our success. Others are internal areas where we have more control. In both cases, we struggle to connect the dots - to trace any causality from the circumstance, to our outcomes.
Some beliefs are more relevant than others - it is context-dependent. Here is an example of a worksheet that could solicit beliefs for a simple product business.

Context-specific areas where beliefs can be mined
External Areas (more outside our control)
- Macro-economic trends: “Are we headed for a soft landing?”
- Category lifecycle: “Is the category saturated and peaking?”
- Market size: “Is the market still growing?”
- Market needs: “What needs to be added to the value proposition?”
- Technology trends: “What is the impact of AI on our product?”
- Buyer preferences: “Are buyers more cost-conscious than last year?”
- Segment size: “Is this new segment worth emphasizing?”
- Consumer trends: “What does Gen Z think?”
Internal Areas (more under our control)
- Competitive landscape: “Is Company X a threat?”
- Product lifecycle: “Is growth waning… are we mature now?”
- Internal capabilities: “What business capabilities are we lacking?”
- Interface performance: “What business capabilities are underperforming?”
Some of these beliefs will be more relevant than others. Identify that most important ones as “core” to the strategic conversation.
When these opinions are expressed as beliefs, we use a syntax that let us predict whether the statement will be true or false, while acknowledging the uncertainty:
“We believe that <this thing> will happen, to <this degree>.”
When people collaborate and debate these opinions, they can work towards a shared understanding of the environment around them. Differences in opinion are inevitable. If the belief can be crisply expressed as something that can either turn out to be true or false in the future, we can steer the conversation to probabilities.
Living in a probabilistic world
People will have differing opinions on these topics and these are healthy debates. We can keep it healthy and productive by acknowledging uncertainty, and expressing the differences in opinion as differing probabilities (i.e. that the belief will be true).
How can we explore these differences in a way that nudges us toward a shared understanding?
Consider breaking the discussion for a belief into three parts:
- A dialog that explores the range of opinions for the area (e.g. for category lifecycle, or technology trends) and drafts belief statement(s)
- A discussion of the relevance, significance, or importance of the belief statement, with respect to the ongoing strategy conversations
- An anonymous, asynchronous survey or poll-like activity that asks a group of people to provide their opinion on the probability that the belief statement will be true, with rationale (and, optionally, links to supporting evidence).
For the second step, a Belief Map offers a visual way to compile these opinions of relevance, along with a self-assessment of the contributor’s relative expertise or knowledge of the situation.

When a cluster appears in the upper right, then credible people are suggesting the belief is very relevant, for your context. In these cases, a particular belief is worthy of some dialog about its likelihood, or probability of occurrence.
The next step is to get a shared understanding of the likelihood that the belief is true. Sparking discussions around, “What conditions would have to exist, for this to be true?” can get a healthy dialog started.
Since a belief is a forecast about the (unknowable) future, there will (still) be differences in opinion after the dialog. Instead of trying to gain consensus on the likelihood, collect the range of opinions, using a technique like the Mini-Delphi Method.
- Refine the belief statement to confirm that it could be resolved as true or false (someday)
- Select a group of people to participate - this could be the same group that were involved in the dialogs, or it can be expanded to solicit additional opinions
- Ask each participant for their forecast, as a probability, with supporting rationale. This should be done anonymously, and can be done asynchronously.
- After the last input is collected, compile the probabilities into a range.
- Share all responses back out to the participants and ask for feedback on others’ responses.
- Collect refined responses into a summary, including a single, baselined probability for the belief.
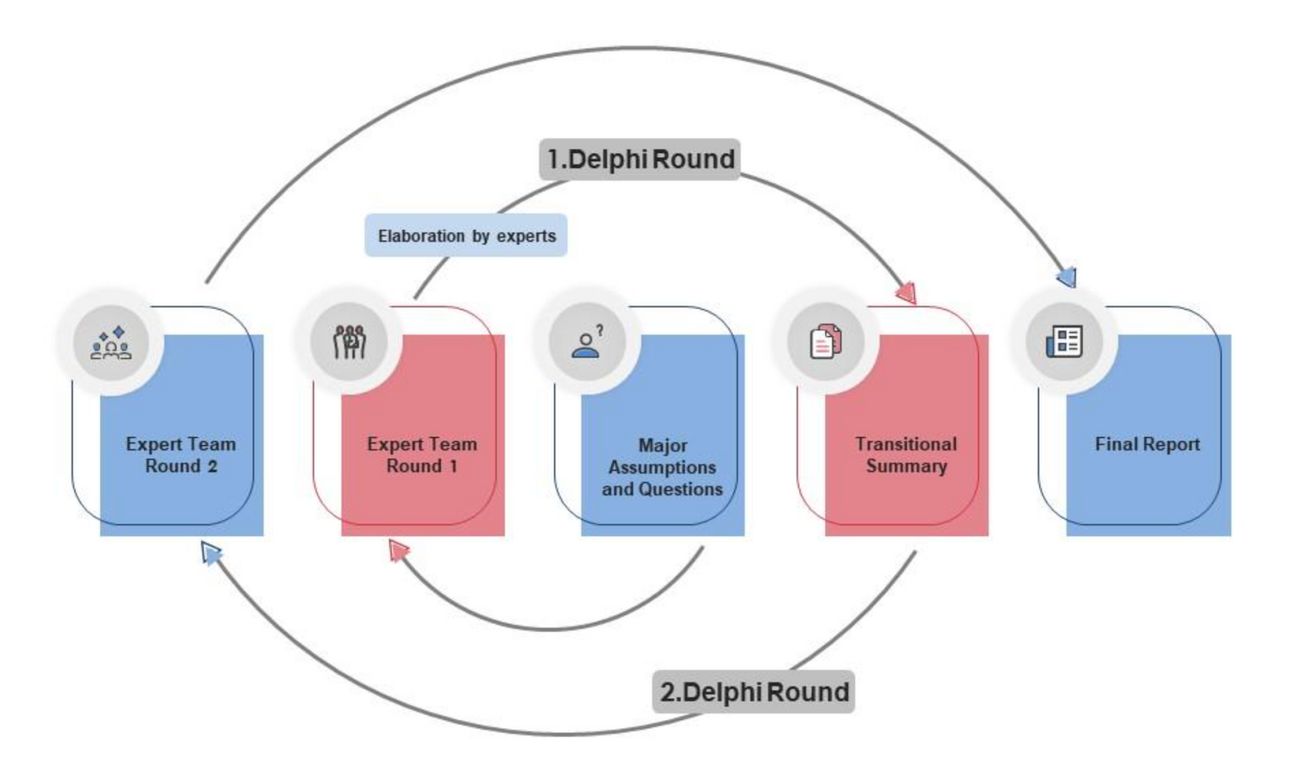
With this exercise, a leadership team can set baseline probabilities, for this point in time, for each belief.
Over time, however, our opinions will change.
This is arguably the most valuable reason for documenting beliefs: When new information comes to light (i.e. we learn something new), we can revisit our probabilities, and then reshape our vision and strategic choices.
This revisit and challenge of our beliefs can be done reactively or proactively (or both!). To be proactive, a leadership group can set “trip-wires” for each belief, triggered when some observed measurement reaches a particular value. In this way, a belief can be proactively challenged.
Alternatively, beliefs can be periodically revisited (say, annually), and a belief portfolio review activity can be conducted.
Sometimes, though, events happen (out of the blue) that get us to question our core beliefs. How should we react in this situation?
Challenging beliefs
The world around us is constantly changing. The competitive marketplace can be turned upside-down. Consumers are fickle. Economic conditions can turn on a dime. Usually, we think of these events as things that are happening “to us”.
But at the same time, we are constantly seeking to reduce uncertainty through our own actions and efforts. Running experiments. Using discovery to buy information. Delivering incrementally to drive desired outcomes. This creates events that are happening “through us.”
When insights emerge, these events can (and should) invite us to challenge our beliefs.
So… when you get an insight (hooray!), what are you supposed to do?
The question we need to ask is:
“What is the impact of this new insight on our belief(s)?”
Since we have expressed our beliefs in probabilistic terms, we can bring some Bayesian thinking into our approach for answering this question. Bayes’ Theorem helps us think about how to apply the new information (or insights), to challenge a belief.
“As an empirical matter, we all have beliefs and biases, forged from some combination of our experiences, our values, our knowledge, and perhaps our political or professional agenda. One of the nice characteristics of the Bayesian perspective is that, in explicitly acknowledging that we have prior beliefs that affect how we interpret new evidence, it provides for a very good description of how we react to the changes in our world.” - Nate Silver, “The SIgnal and the Noise”
We can apply Bayesian thinking at two levels of rigor here. At a simple level, it invites us to revisit our prior probabilities for a belief, when new information becomes available. This implies, of course, that we took the time to capture a forecast for the belief up front.
Nate Silver says, “This is perhaps the easiest Bayesian principle to apply: make a lot of forecasts. You may not want to stake your company or your livelihood on them, especially at first. But it’s the only way to get better.”
The second, more complicated (and less common) way to apply Bayesian thinking, is to actually do the math.
Let’s run through a couple examples, to show how it can be used to translate insights (new information) into updated beliefs (new probabilities).
Here’s a simple illustration, loosely based on the saying, “Where there is smoke, there is fire…”.

A Bayesian assessment would follow like this:
- Review the “prior” probability. Let’s say that when we constructed the belief (that essentially says we’re not currently worried about a big fire), we set the probability of a big fire at 1%.
- Assess the overall probability for the event, based on the new information. This is called the “marginalization” in Bayesian terms. Here we would acknowledge that smoke can result from sources other than fires, and we put the probability of seeing smoke at 10% overall.
- Next (and this is the strange one…), we work the “backward” direction to assess the “likelihood” that the event would happen if you assume that the belief is true. So in this case, if we actually do have a fire on our hands, what is the probability that there will be smoke? We’ll say 90%.
- Apply Bayes Theorem to calculate a new probability for the belief, given the new information conveyed through the event. So the calculated probability of a fire, given the fact that we see smoke, is 9%.
So through this exercise, we took the new information (“we see smoke”) and might revise our probability of a fire from 1% to 9%.
We say “might” because the math is just a tool. The choice to change a probability in the belief portfolio should always fall to the judgment of the leaders (not the math), but this exercise can structure and support a “belief challenging” activity, in response to new information and insights.
For example, the calculated update can be circulated amongst the leadership group for comment, discussion, and dialog. Do people intuitively agree with the result? What does the discussion reveal?
Again, this is only possible if we collect the initial forecast on the belief. Nate Silver says, “Bayes’ Theorem requires us to state - explicitly - how likely we believe an event is to occur before we begin to weigh the evidence.”
Here’s a more thorough description of the conditional probabilities in Bayes Theorem.

Now let’s run through two more examples, closer to our domain.
- We have a belief that Competitor A is not much of a threat to our market share, but then we get an event where their sales double in the last quarter!
- We have a belief that Project X will complete on-time and on-budget, but then we get an event where the project fails to hit a critical milestone!
These are two classic cases where, as a leader, you might just “stick your head in the sand”. Can Bayesian thinking help? Let’s do the math and see what we think.
For the first example, we:
- Start with a belief that it is only 30% likely that Competitor A is a direct threat.
- Evaluate the probability of the event happening, in two scenarios: one where the belief is true (they are threatening us) and the other, where they are not directly competing with us. We decide that it is 20% likely that this sales jump would happen if they were encroaching on us, and only 5% likely that the sales jump would happen if they were not threatening us.
- We apply Bayes Theorem to find that the calculated probability that Competitor A is a threat, given their recent sales jump, is 63%.
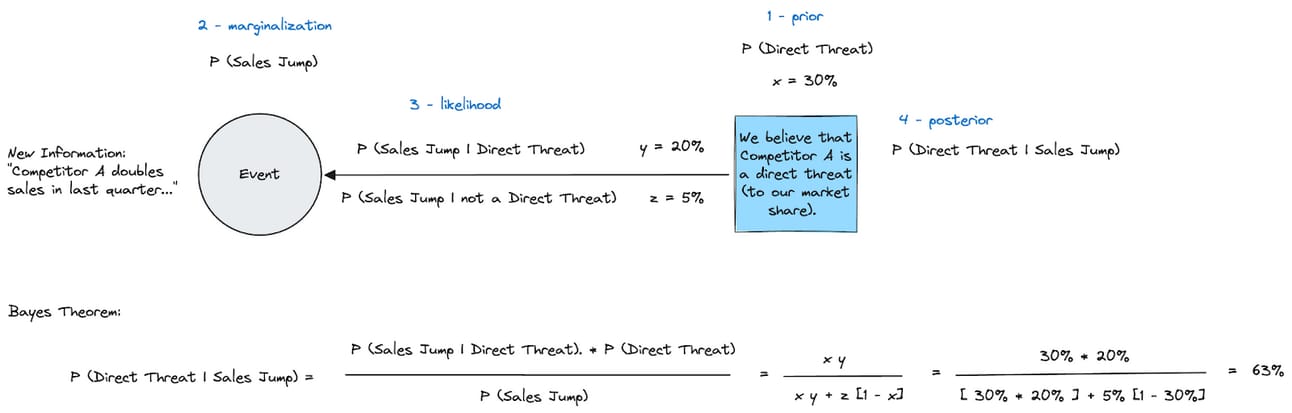
So the recent event, after analysis, suggests that we should challenge our belief on whether they are a direct threat (to our customers, to our market space, etc.).
For the second example, we:
- Start with a belief that it is 80% likely that Project X is on track.
- Evaluate the probability of the event happening, in two scenarios: one where the belief is true (the project is healthy and on track) and the other, where it is not. We decide that it is 5% likely that this missed milestone would happen if the project was still on track, and 60% likely that the missed milestone would happen if the project is not on track to finish on time and on budget.
- We apply Bayes Theorem to find that the calculated probability that Project X is on track, given the missed milestone, is 25%.
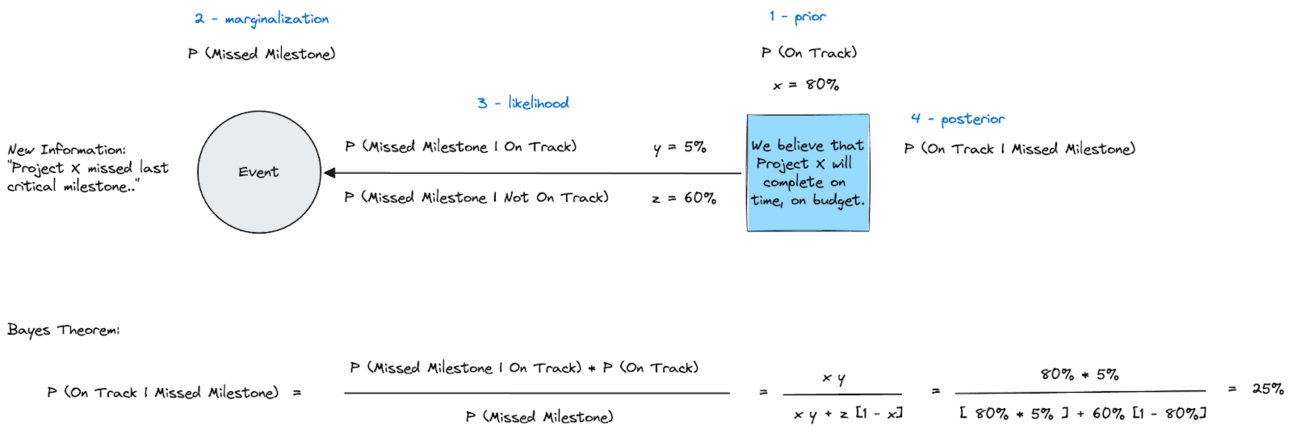
So the recent event, after analysis, suggests that we should challenge our belief on whether the project is on track, since the drop from 80% to 25% is pretty dramatic.
While this internal project example is relatable, it is better to apply this to the harder-to-assess external areas listed earlier, since those beliefs tend to be challenged the least, and the challenges (when they happen) have little structure today.
Then what?
In cases where the leadership team arrives at big changes in the probabilities, there may be strategic implications. Beliefs form the foundation for strategic choices, which drive investment decisions. Tracing relationships between (changing) beliefs and these investments can open the door to better risk assessments across the active work.
If we used to think X was 90% likely, and are currently executing in a strategic direction, fueled by these strategic investments, then where are we at risk if we now think X is only 40% likely? New risks will surface, and can be managed. In extreme cases, projects can be canceled, and investments can be reclaimed, repurposed, and reallocated. This is what strategic agility looks like.
Hopefully this article helps you see the potential value of capturing a belief portfolio, and carving out time for belief challenging, with or without Bayesian analysis. Bringing these invisible mental models (i.e. beliefs) to the surface enables us to manage what was previously unmanageable, and, ultimately, to make smarter decisions.