Exploring Causality and Complexity in Strategy
On this learning journey with the Uncertainty Project, there have been a couple “light-bulb” moments that have changed my perspective significantly. The most recent one is how complexity impacts our ability to understand causality.
I found that these topics of causality and complexity get very deep, very fast. The mathematics, algorithms, and theories behind it underpin much of modern artificial intelligence. And the frameworks around it are more likely to cite Greek philosophers than business thinkers. So this newsletter is going to be a view from the shallow end of a deep pool.
But it feels significant! The idea of causality is something that we usually take for granted, and, honestly, don’t spend much time questioning in our day-to-day use of it. The idea of causality matters greatly, though, since as we apply our agency (as individuals, as organizations, and businesses) to take action, we base our choices on where we believe we can cause good things to happen.
Getting a deeper understanding of causality can also help us see emerging boundaries between ourselves and our new AI assistants. Seeing how algorithms can (and can’t) bring causality into our analysis of data reminds us that the final judgment is still a job best left to humans.
As Yann LeCun, chief scientist at Facebook’s Artificial Intelligence Research lab explained in “Artificial Intelligence - How We Help Machines Learn”:
“Humans possess a breadth of skills far beyond that of any machine yet invented. We rely, at a minimum, on four interconnected capabilities to successfully navigate the world:
- to perceive and categorize things around us;
- to contextualize those things for understanding and learning;
- to be able to make predictions based on past experience and present circumstances and
- to make plans based on all of the above.
Add these together, and you get common sense — those functions you need to survive and thrive.“
Most of the time, we associate strategic decision making with navigation capabilities 3 and 4 above, and less so about navigation capabilities 1 and 2.
But “to be able to make predictions” (capability 3) requires an understanding of causality. Can our categorization (capability 1) and our understanding of context (capability 2) give us any insight into where, when, and how we can apply causality to our decision making?
It can, but we need to leverage some new frames - as windows into our world - that shift more attention to our problem domains, our local context, and the role causality can play in our analysis of data.
Domains
When we shift from being data-centric to being decision-centric, we frame a situation or challenge first, and only then bring in the analysis and data. Part of that exploration of the situation or challenge is to explore patterns of causality: finding specific examples of it (e.g. expressed in causal diagrams), and comparing relative strengths of the causal relationships.
Think about it. When we set out to design a strategy, we consider various “levers to pull”, that will put the wheels in motion towards some desired outcomes. But this “lever” metaphor implies a cause-and-effect - it implies that causality is both understandable and understood in our context. Beyond that, we tend to view these “levers” as the triggers of perfect machines - we talk with certainty about the effects we can cause with them - not as the handles of slot machines, spinning wheels of uncertainty. And our brains conspire against us in this moment, happy to move forward with the first solid idea for a “lever” that comes to mind!
How can we follow those same four navigation capabilities in our strategic decision making, with a deeper understanding of causality?
- “Categorize” the situation, domain, and/or challenge (hint: it’s often “complex”)
- Focus “understanding and learning” on your local context first (instead of automatically looking outside for answers or options)
- If you find yourself in a complex domain, “predictions” are not possible, and the inherent uncertainty should drive us to a more experimental approach, seeking patterns
- To get to “plans”, bring new approaches, fit for the context:
- Explore existing (and new) constraints to influence the formation of coherence
- Encourage divergence when drawing causal diagrams (tease out causal hypotheses from patterns or correlations)
- Acknowledge uncertainty with probabilistic thinking and hypothesis building
- Shorten the feedback loops, to engage the causal inference engine
Let’s start with how categorization of our situation or challenge (i.e. problem space) can help steer how we discuss causality in strategic decision making. You’ve likely already come across the work of Dave Snowden, but if not, here’s a great introduction:
"Snowden's framework is called the Cynefin framework - pronounced "Ken-ev-in" (it's Welsh, like Snowden, and a little quirky). The core distinction Snowden makes is between those issues or events that can be generally predicted and those that cannot be. Those things that can be generally predicted live in the land of the probable, where we can make a high-reliability guess about the future from observing and understanding the past. Those things that cannot be reliably predicted live in the world of the possible, where the future rests on too many unknown variables to predict from the past. This is a powerful way to understand your decision making, and it's one we should think a lot more about." - from "Simple Habits for Complex Times" by Jennifer Garvey Berger and Keith Johnston.
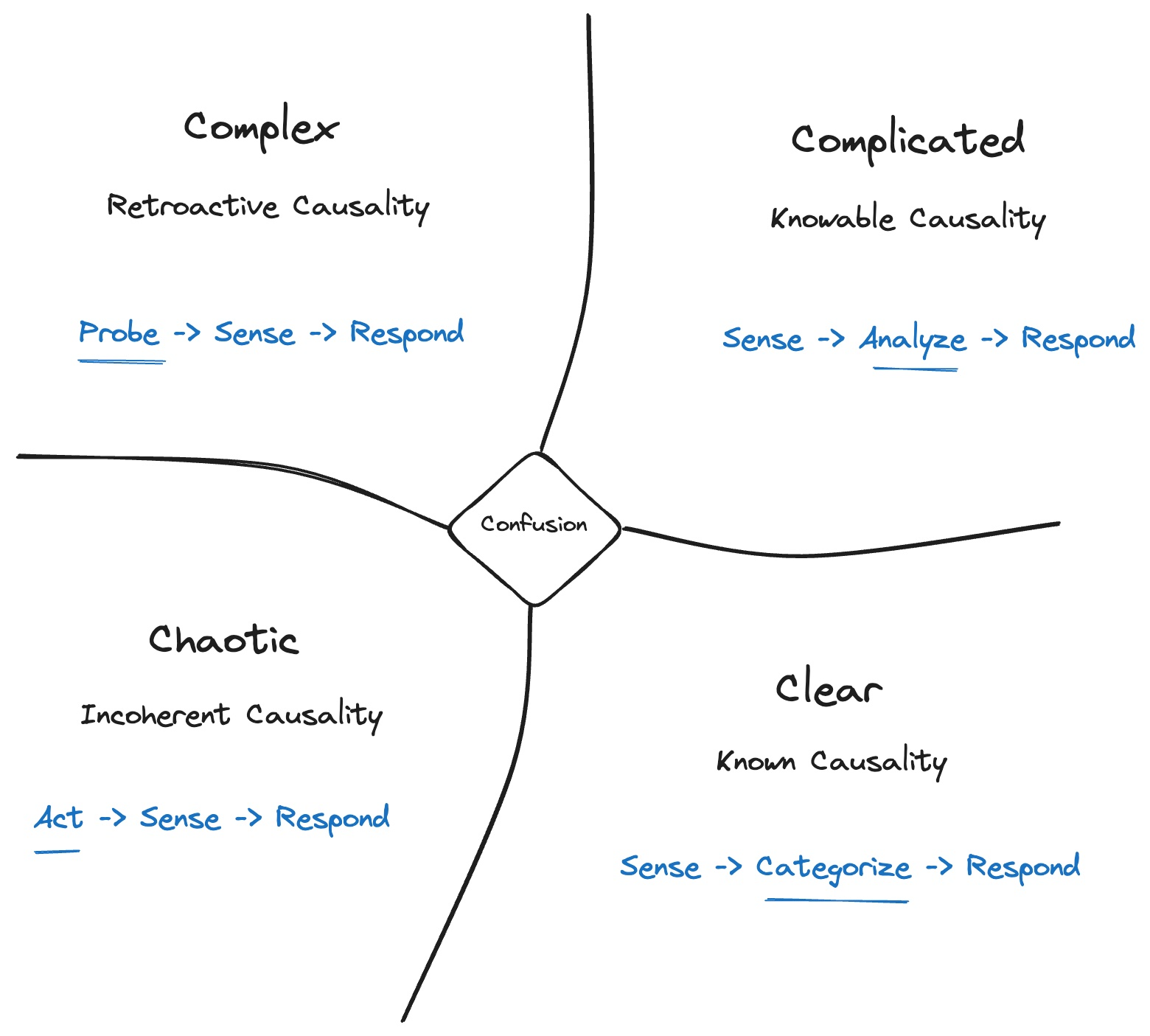
Using Cynefin to categorize the challenges is a good way to begin strategy design, to develop situational awareness.
The Cynefin domains are differentiated by causal understanding:
- Clear: Causality is understood, so you better understand it before setting strategic direction
- Complicated: Causality is can be known via analysis, so it’s usually worth dedicating some time analyzing things
- Complex: Causality is emergent, so probe and analyze before responding with big plans
- Chaos: No chance at finding causality any time soon, so bias for action, not strategy
These days, many modern business challenges put us in the complex domain. In last week’s newsletter, our colleague Marco Valente nicely summarized how causality works in a complex domain:
“In Complex systems, the links of causality and the corresponding understanding of what happened can only be seen in retrospect. We can fully make sense only by looking back at what happened; the system does not show itself its initial conditions fully, because information is decentralized, traveling fast, and variables keep evolving, and hence even less so can we predict what will the system do next. Still, the system can show regularity in its patterns, and inclinations to produce certain outcomes (what Dave Snowden calls dispositionalities). When you want to devise a path forward in a complex system there is no way to fully understand its patterns until you start playing around and ‘poking’ the system (because, e.g., only then you will see how rigid its resistance to change is); later you sense the system and respond adequately in a constantly dynamic relationship.”
So without this categorization (in navigation capability 1), it’s easy to make some bad fundamental assumptions in strategy design:
- Assuming causality can be understood/anticipated when it can’t (i.e. complex domains)
- Assuming a lever will, with 100% certainty, lead to a desired effect
- Assuming the effect will, with 100% certainty, lead to a desired goal
- Assuming that your situational and contextual domain is still the same as it was the last time you designed strategy
That last one is especially dangerous, as Snowden explains,
“It’s important to remember that best practice is, by definition, past practice. Using best practices is common, and often appropriate, in simple contexts. Difficulties arise, however, if staff members are discouraged from bucking the process even when it’s not working anymore. Since hindsight no longer leads to foresight after a shift in context, a corresponding change in management style may be called for.” - David Snowden & Mary Boone, “A Leader’s Framework for Decision Making”
Observing your decision making (within your decision architecture) is a good way to sense whether the domain is shifting beneath your feet:
“Reaching decisions in the complicated domain can often take a lot of time, and there is always a trade-off between finding the right answer and simply making a decision. When the right answer is elusive, however, and you must base your decision on incomplete data, your situation is probably complex rather than complicated.” - David Snowden & Mary Boone, “A Leader’s Framework for Decision Making”
Context
So if we can’t talk about causality in our complex domains, what can we talk about? What can we use as “levers” to actively probe and navigate?
Here we shift to navigation capability 2, where we seek a rich understanding of our context.
Alicia Juarrero, in her book “Context Changes Everything”, sought to explain how a context can exert influence on its component parts. She deliberately avoided the term “cause” to describe its impact, saying that causality had too much “baggage”. Instead she uses the concept of constraints to describe this influence.
She offers a great thesaurus-worthy definition of constraints:
“Constraints are entities, processes, events, relations, or conditions that raise or lower barriers to energy flow withour directly transferring kinetic energy. Constraints bring about effects by making available, structuring, channelling, facilitating, or impeding energy flow. Gradients and polarities, for example, are constraints; others include catalysts and feedback loops, recursion, iteration, buffers, affordances, schedules, codes, rules and regulations, heuristics, conceptual frameworks, ethical values and cultural norms, scaffolds, isolation, sedimentation and entrenchment, and bias and noise, among many others.”
More importantly, she describes how constraints create coherence within the complexity:
“Constrained interactions leave a mark. They transform disparate manys into coherent and interdependent Ones. Constrained interactions, that is, irreversibly weave separate entities into emergent and meaningful coherent wholes. In doing so, they create and transmit novel information. That information is embodied in the coordination patterns formed by, and embedded in, context. The central question is: What changes reversible bumping and jostling into interactions that leave a mark - that create order, structure, and information? The answer is… context-dependent constraints.” - Alicia Juarrero, “Context Changes Everything” (2023)
She argues that “interlocked interdependencies generated by constraints are the ground of coherent dynamics and their emergent properties.” She builds a case that this kind of influence-on-effects (I’m not calling it causality…lol) can pass “bottom up, and top down, from parts to whole, and whole to parts.”
So the takeaway is that context-specific constraints are something we can actively manage, in a complex domain.
One example of how constraints surface in a business setting (and can also improve visibility of different domains), is Geoffrey Moore’s “Zone-to-Win” framework. To apply his concepts from the seminal book, “Escape Velocity”, he set out to devise a framework that can balance the need for investment in both sustaining and disruptive innovation.
What’s interesting is that each zone typically operates in a different Cynefin domain. I say typically, because - you guessed it - the contexts will vary. But what matters is that, in a context, these kinds of constraints (e.g. zones) can steer a different strategic approach into each zone:
- Stabilize the Performance Zone to behave closer to a Clear domain
- Drive the Productivity Zone as a Complicated domain
- Operate the Incubation Zone to run safe-to-fail experiments as a Complex domain
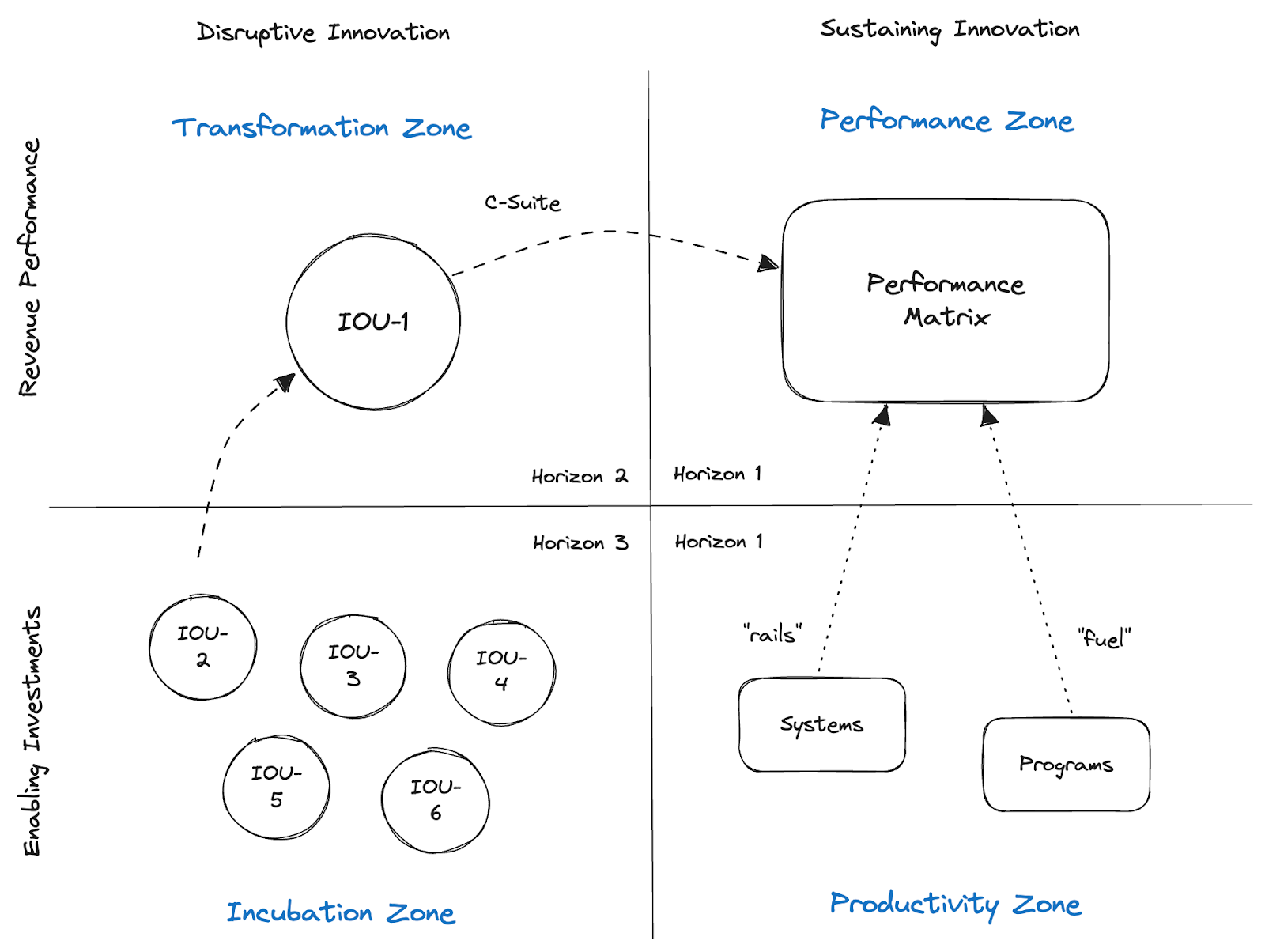
Let’s zero in on the Productivity Zone a bit, and say (hypothetically) that it has been categorized by leadership as a Complicated domain, that is, a problem space where causality can be understood, with some analysis.
In the Productivity Zone, according to Moore, leaders enhance supporting Systems (e.g. IT systems) and/or seek to design Programs (e.g. marketing programs or product launches) that enable teams to hit performance targets (in the Performance Zone). Your list of active projects and initiatives lives here (a list which is probably too long, btw…).
As leaders ideate in the Productivity Zone, they can apply their knowledge of the past to inform suggestions for the future, if it's deemed to be a Complicated domain. That’s how causality is applied to help us explore various strategic levers for change.
When it's Complicated, we might analyze our way to some best choices, make decisions, and move forward with plans. When the world is changing quickly around us, though, we might have to admit that our efforts at improving productivity have shifted into a Complex domain. In this situation, we need more of an experimental mindset that seeks shorter feedback loops to “probe-sense-respond” and look for emergent patterns.
In both cases, it’s good for a leadership team to use visual aids like Causal Decision Diagrams to help them develop a shared understanding.
“Simply drawing good collaborative pictures of our ‘common sense’ understanding of causation is such a great step forward that we shouldn’t allow formal theories of causation to get in the way. This can be dangerous though, so an important direction for DI (decision intelligence) is to translate formal causation theory into a form that can be used for non-technical practitioners.” - Lorien Pratt, “Link” (2019)
In a Complex domain, you’ll want to encourage divergent thinking, and explore many angles for probing the system. In a Complicated domain, it’s more realistic to leverage observational data sets to build causal arguments (in advance) for some choices.
Data
These data sets (of past observational data) can expose statistical correlations, but won’t offer support for causal arguments on their own. But when the causal diagrams are combined with the data sets (and some new theories on causality), it can offer data-driven support for decision-driven causal arguments.
This marriage of causality and statistics was the life’s work of Judea Pearl, author of “The Book of Why” <link>. He found ways to apply statistical techniques to explore and support hypotheses with a combination of causal diagrams and data.
He also offers a beautiful description of causality, as “listening”:
When Y “listens” to X, then there is a causal relationship from X -> Y
Which nicely evokes that the influence is pulled, not pushed.
Pearl summarized his process flow for causal inference in this diagram:

If you are a leader exploring a challenge categorized as a Complicated domain, then as you look for “levers to pull”, you could capture them as causal diagrams. This might open the door to using the “inference engine”, to enhance your analysis:
- Capture your assumptions that serve as the foundation for the causal diagram
- Complete the causal model, to show relationships between key variables (who “listens” to whom…)
- Test the implications of the model by looking for patterns and dependencies in the data
- Devise a scientific question as a query, using Pearl’s new causal vocabulary
- Explore whether the query can be answered, given the causal model and the data set
- If it can, provide the statistical answer, using the data
- If it can’t, refine the model with new assumptions or different variables (and try again)
Many of his examples came from medical contexts, where, for example, the causal relationship between symptoms and a disease are being investigated. In our business contexts, the analogous case might be to explore causal relationships between various contributing factors (symptoms) and the key challenge (disease).
This hints at how sophisticated data analysis and Pearl’s causal algebra can be leveraged in a strategic decision architecture. Notice how the flow leads with questions and assumptions, and uses the data to serve the pre-existing hypothesis.
Also consider how the flow could help answer retrospective questions about your decision architecture, like, “Did my decision actually make a difference on these outcomes?” (i.e. was a contributing cause to the result). It might be useful to bring more rigor to your outcome fielding.
This article surveys just the tip of the iceberg, on how a decision-centric approach to strategy can more thoughtfully apply concepts of causality, build around known constraints in the context, and use data to support causal hypotheses and build confidence with statistical significance.
I mentioned that these new concepts hit me like a flash of light, but honestly, it’s still just a dim 40-watt bulb.
Join the conversation here at The Uncertainty Project, and let’s work together build a spotlight!